In recent years, the computer science-oriented machine learning paradigm, mainly based on artificial neural networks, and the neuroscience-oriented neuromorphic computing paradigm, mainly based on spiking neural networks, have been widely studied and applied in intelligent systems. Each paradigm has its advantages and disadvantages in terms of applicable feature types, accuracy, robustness, and computational cost, and a single paradigm alone is insufficient to support the exploration of artificial general intelligence. Meanwhile, hybrid neuromorphic computing, which supports both types of intelligent models, provides sufficient flexibility for cross-paradigm modeling and is one of the most promising approaches for the development of artificial general intelligence. Recently, research on hybrid neuromorphic computing chips has been growing, with notable examples such as Tsinghua University’s “Tianji Chip,” Heidelberg University’s BrainScaleS 2, the University of Manchester’s SpiNNaker 2, and Intel’s Loihi 2. However, these works have mainly focused on the chip design itself and have not demonstrated support for large-scale workloads of neuromorphic computing systems, hindering the practical applications of hybrid neuromorphic computing chips.
To address the above issues, the center has built a multi-granularity hybrid neuromorphic computing system based on the self-developed “Tianji Chip.” Leveraging the highly scalable and decentralized architecture of the multi-core chip, this system offers great flexibility in hardware integration and granularity, making it adaptable to different scenarios. For instance, a 4-chip board can be used for embedded neuromorphic computing platforms, while a 25-chip board can be used for neuromorphic computing server platforms. Furthermore, a software toolchain, including a system simulator and compiler, has been developed to achieve flexible configuration of the system and efficient deployment of models. Based on the constructed hybrid neuromorphic computing system, researchers have tested various forms of computing boards and servers, demonstrating the superior performance of the Tianji neuromorphic computing system. This provides a powerful and efficient hardware and software platform for exploring artificial general intelligence-related models in the future.
成果发表于2023年《Science China Information Sciences》
近年来,以人工神经网络为主要模型的计算机科学导向机器学习范式和以脉冲神经网络为主要模型的脑科学导向神经形态计算模型被广泛研究并应用于智能系统。二者在适用特征类型、准确率、鲁棒性和计算代价等方面各有优劣势,单一范式均不足以支撑人工通用智能的探索。同时支持上述两类智能模型的混合范式类脑计算为跨范式建模提供了足够的灵活性,是发展人工通用智能最有潜力的技术途径之一。近期混合范式类脑计算芯片的研究呈增长趋势,主要代表有清华大学的“天机芯”、海德堡大学的BrainScaleS 2、曼彻斯特大学的SpiNNaker 2、英特尔的Loihi 2等。然而这些工作主要聚焦芯片设计本身,并未演示支持大规模负载的类脑计算系统,阻碍了混合范式类脑计算芯片在实际中的应用。
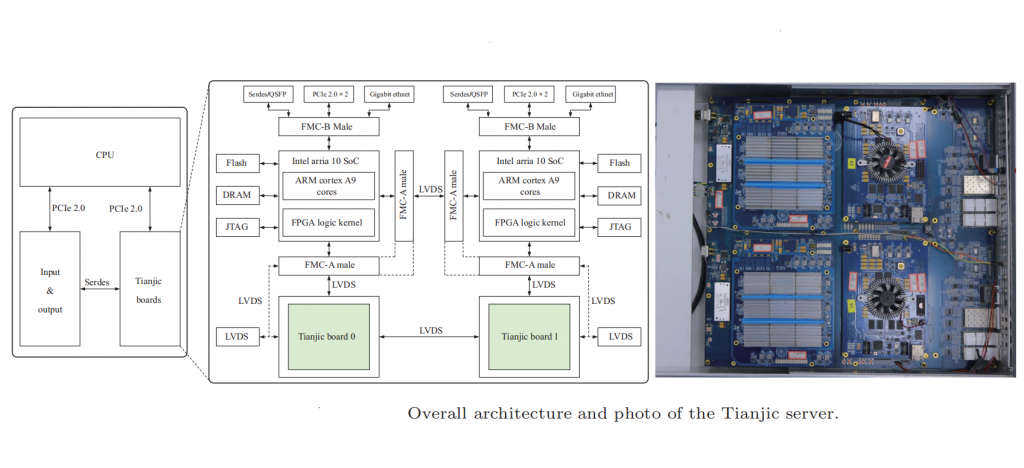
针对上述问题,中心基于自研的混合范式类脑计算芯片—“天机芯”构建了多粒度混合范式类脑计算系统。凭借众核去中心化架构的超强扩展性,该系统的硬件集成形态和粒度具有很强的灵活性,通过改变计算板卡集成的芯片数量和连接拓扑可以适用于不同场景,其中4芯片板卡可用于嵌入式类脑计算平台而25芯片板卡可用于类脑计算服务器平台。进一步开发了包含系统仿真器和编译器的软件工具链,实现系统的灵活配置和模型的高效部署。基于所构建的混合范式类脑计算系统,研究者们测试了各种形态的计算板卡和服务器,展示了天机类脑计算系统的优越性能,为未来探索人工通用智能相关模型提供了强大高效的软硬件平台。